Virtual digital twin of mechanical ventilation
This task is dedicated to developing a virtual digital twin for critically ill patients, a process that involves numerous sequential decisions made every hour. This use case is particularly suited for reinforcement learning, which will be employed to determine the optimal treatment choices tailored to the specific conditions of individual patients at any given moment.
A primary focus will be optimizing mechanical ventilation settings for treating respiratory failure. Crucial to this use case is the leverage of the MIMIC-EU dataset, as referenced in use case 1. The integration of this diverse and extensive dataset is key in customizing treatment decisions for a wide variety of patient profiles, thereby enhancing the generalizability of the developed models.
Furthermore, supervised machine learning will be utilized to predict and prevent the need for reintubation and to guide clinicians on data collection for improved predictive accuracy. The outcomes of this work will be disseminated through scientific literature and a pre-print on the knowledge platform, facilitating the rapid exchange of best clinical practices across Europe.
This task includes:
T6.3.1
Utilise the procedures, processes and templates developed by experts from the ELSI framework task (WP3) and implemented in WP4 to gain access to data from the task contributors for federated analysis.
T6.3.2
Develop and test a reinforcement learning model to optimise mechanical ventilator settings, taking into account sex differences, physiologic differences due to ethnic background, body mass index and comorbidities.
T6.3.3
Develop guidance, training, and education materials on the development, testing and validation of reinforcement learning models in a federated setting for publication through the knowledge platform.
Use case leader
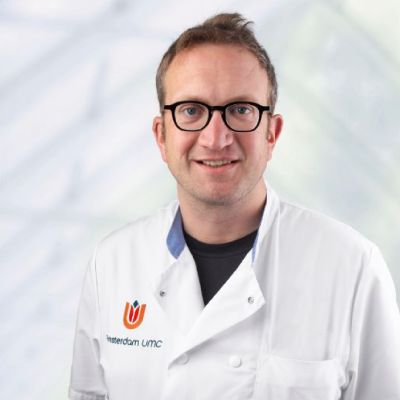