Early detection of organ failure
This task aims to demonstrate the development and deployment for clinical evaluation of machine learning models to predict organ failure based on time series data. The high availability of time series data is a unique selling point for ICU data. This use case will demonstrate how that data can be leveraged. To do so, this task will focus on the highly clinically relevant problem of (multi) organ failure. The premise being that if organ failure is predicted when still reversible, health outcomes would improve and clinical workflow would benefit as timely, planned interventions are more beneficial and pose less burden on health care workers.
This task includes:
T6.2.1
Select organ systems based on availability of data and outcome information from MIMIC-EU (T6.1) utilising the procedures, processes and templates developed by experts from the ELSI framework task (WP3) and implemented in WP4 to gain access to data for federated analysis.
T6.2.2
Establish a data adjudication committee to provide guidance on quality control and facilitate harmonised annotation of health outcomes, including organ failure of the following organ systems: kidneys, brain, liver, cardiovascular in collaboration with the Data Provider Support working group.
T6.2.3
Develop, test, and validate machine learning models to predict organ failure of the specific organ system, taking into account regional differences, sex differences and minorities.
T6.2.4
Design a clinical evaluation study for the machine learning systems.
T6.2.5
Deploy the machine learning systems for clinical evaluation at participating institutions.
Use case leader
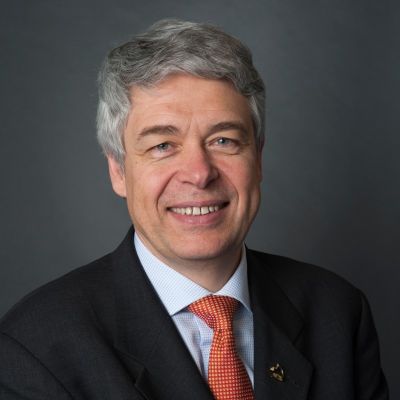